How to buy and sell AI in healthcare? Not easy. – Healthcare Blog

Matthew Holt
Not long ago, you could create one of one of the maps of healthcare or digital health, and it was roughly correct. I did my own 2.0 days of health, including the old subcategory of “Rebel Alliance of New Provider Technology” and “Borders of Patient Empowerment Technology”
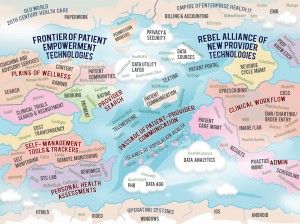
But, the simple days of matching SaaS products with expected users and distinguishing them from other users. The map has been disrupted by a hurricane that generates AI and has put the industry in a state of chaos.
Over the past few months, I've been trying to figure out who will do what in AI Health Tech. I have had many formal and informal conversations, read a lot, and have attended three meetings over the past few months, all focusing on this topic. Obviously, no one has a good answer.
Of course, this hasn't stopped people from trying to draw such maps from Protege. As you know, there are hundreds of companies building the first AI products for every aspect of the chain of healthcare value (or lacking it!).
But this time it's different. It is not clear that AI will stop at the user's boundaries and even have well-defined features. It is not even clear that there will be an “artificial intelligence of health technology” field.
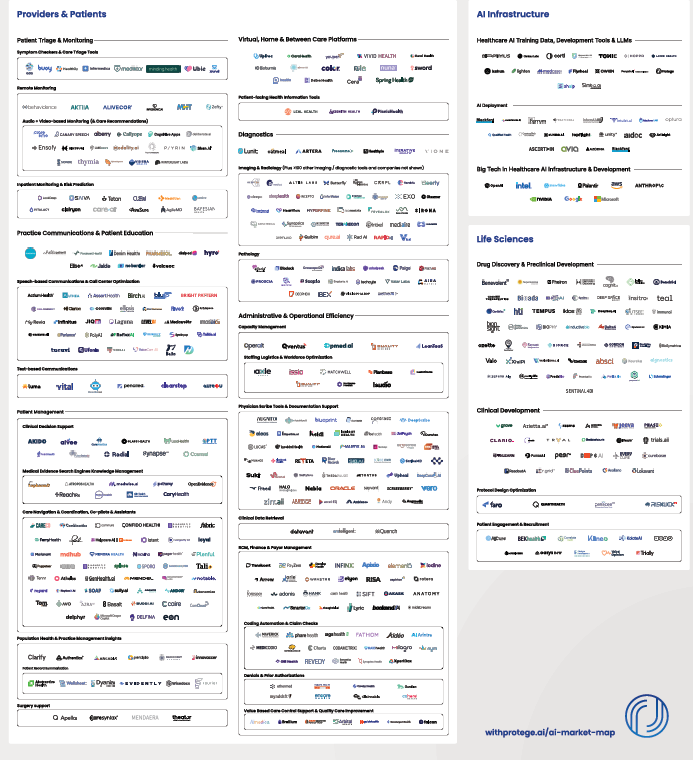
This is a multi-dimensional problem.
The main AI LLMS -Chatgpt (OpenAi/Microsoft), Gemini (Google/Alphabet) Claude (Anthropic/Amazon), Grok (X/Twitter), Lama (Meta/Facebook) – all do incredible work in healthcare as well as outside. They can now create movies, music, images in any language you like, and get better and better.
They are excellent in explanation and summary. The other day, I literally dumped a rather incomprehensible 26-page intensive CMS RFI document into Chatgpt, and after a few seconds it told me what they asked for and what they actually wanted (that unwritten subtext). The CMS officials who wrote it were impressed and they were not allowed to use it. If I want to help CMS, I will also write a response for me.
Big LLM is also developing the “agent” function. In other words, they are able to perform multi-step business and human processes.
Currently, healthcare professionals and patients are used directly to abstract, communicate and accompany. They are increasingly used in diagnosis, coaching and treatment. Of course, many healthcare organizations use them directly for process redesign.
Meanwhile, the core moderator of health care is the EMR used by providers, the largest of which are epic. Epic has its own AI gaming relationship with Microsoft and has its own strong relationship with Openai — or at least as strong as investing $13 billion in a nonprofit, which will make your relationship. EPICs are now all using Microsoft's AI in Note Summaries, Dister Communications, etc., and use the nuanced DAX (DAX) of Microsoft's subsidiary. Epic also has a relationship with DAX competitor Abridge
But that doesn't necessarily have enough, and Epic is clearly building its own AI capabilities. In a good review Healthy today John Lee breaks down Epic's non-trivial use of AI in its clincal workflow:
- Now, the platform provides tools to reorganize texts to understand readability, generate concise, patient-friendly summary, hospital course summary, discharge instructions, and even convert discrete clinical data into narrative instructions.
- We will be able to automatically automate language in comments (e.g., change “Anaesthesia Abuse” to “Patients with Opium Use Disorders”),
- Even as a doctor, I sometimes have a hard time interpreting shorthand that my colleagues often use. Epic shows how AI translates blunt medical shorthand for “Pod 1 SpCabg. HD Stable.” Amb w asst. –Pure Language: “Day 1 after coronary artery bypass graft surgery after day 1 after OP. Hemodynamic stability. Patients can assist.”
- For nurses, environmental documentation and AI-generated shift notes will be provided, reducing manual input and freeing up time for patient care.
Of course, Epic is not the only EHR (to be honest!). Its competitors won't gain a foothold. Meditech's COO Helen Waters conducted extensive interviews with Histalk. I've paid particular attention to her discussion of her work with Google in AI, and I quoted almost everything:
This initial product is built from the BERT language model. It's not necessarily the generated AI, but one of their first large language models. This feature is called Condition Explorer, and it is indeed a leap forward. It intelligently organizes patient information directly from within the chart, and as doctors work in the chart workflow, they can both observe the patient's health vertically through specific conditions and classify this information in a way that clinicians can quickly access relevant information for specific health issues, so that the information is more effective and thus formulate it more effectively.
In addition, with the help of the Centennial AI platform and multiple iterations of Gemini, we have advanced to provide other AI products in the AI generation category, including the narrative of the hospital at the end of the patient at the hospital for discharge. In fact, we generate live courses, which is usually beneficial for the document to not have to start building it on its own.
When nurses switch, we do the same for them. We gave a nurse shift summary, which basically classified the relevant information of the previous shift and saved a lot of time. We are using the Vertex AI platform to do this. Besides everyone else in the sun, we obviously conveyed and brought a live environment transcription feature through AI platforms from numerous vendors, which was also successful for the company.
The concept of Google and partnerships is still strong. Our vision for Expanse Navigator is obvious. LLM’s progress around LLM is continuing, and we see a huge hope that the future of these technologies will help manage administrative burdens and tasks, but also continue to inform capabilities, making clinicians feel strong and confident about the decisions they make.
The voice features in the proxy AI concept are obviously far beyond the scope of environmental transcription, and when you think about how the industry starts with pens, we take them to the keyboard, then we take them to mobile devices and swipe cards with tablets and phones, which is both exciting and ironic. Now, we can go back to voice, and I think it would be pleasant for me as long as it works effectively for clinicians and works effectively.
So if you read (not even between online), but what they say – epic dominates AMC and large nonprofit health systems, while Meditech (EMR for most large for-profit systems like HCA) is building AI for almost all workflows that most clinicians and administrators use.
I came up with many different approaches at a conference hosted by Commure, an AI company centered on catalyst-backed providers. Compperure has gone through many iterations in its 8 years of life, but is now an AI platform that is building multiple products or features. (For more information, here is my interview with CEO Tannay Tandon). These include (so far) management, revenue cycles, inventory and employee tracking, environmental hearing/copying, clinical workflow and clinical summary. You can bet there is more to do with development or acquisition. In addition, Dosure is not only supported in the depths of the general catalyst, but also has partial ownership of HCA, which is also Meditech's largest customer. This means that HCA has to figure out what is doing compared to Meditech.
Finally, there is also a large amount of AI activity within AMCS and among providers, programs and payers. Don't forget that all of these players have made extensive customizations to many of the tools that have been sold by external vendors, such as Epic. They also make their AI vendors “deploy forward” engineers customize their AI tools as their customer’s workflow. But they are building things themselves, too. For example, Stanford University has just released a native product that uses AI to communicate laboratory results to patients. Not purchased from suppliers, but developed in-house using Anthropic's Claude LLM. Dozens of local projects occur in every major healthcare enterprise. All of these data scientists have to stay busy somehow!
So, what does this say for artificial intelligence?
First, it is clear that the current healthcare (EHRS) recording platforms see themselves as large data storage and hope that the AI tools they and their partners develop will take over many of the workflows currently completed by human users.
Second, the technique usually involves water flowing downhill. More and more companies and products eventually become functions of other products and platforms. As you may remember, there was once a separate set of writing software (WordPerfect), presentations (persuasion), spreadsheets (Lotus123), and now there is MS Office and Google Suite. Last month, a company called Brellium raised $16 million from a roughly very clever VC to summarize clinical notes and analyze its compliance. Now watching them prove me wrong, but it seems that everyone and their dog have built AI to summarize and analyze clinical notes? Is it easy to add a compliance analysis? A good bet is that this feature will soon become part of some of the bigger products.
(By the way, one area that might be unique is voice conversation, which now does seem to have a separate set of skills and companies working in it, because interpreting human speech and talking to humans is tricky. Of course, this may be the temporary “moat” that these companies have, which may soon be back in the main LLM).
Meanwhile, Vine Kuraitis, Girish Muralidharan and the late Jody Ranck have just written a 3-part series about how EMR evolved into a larger unified digital health platform, suggesting that the clinical part of EMR will be integrated with all other processes in all other processes. Consider staffing, supply, finance, marketing, etc., of course, the integration between hospitals and ultimately the wider health ecosystem between EMR and medical devices and sensors.
Therefore, integration of datasets may quickly lead to AI-led supersystems in which many decisions are automatically made (such as AI tracking care regimens, as Robbie Pearl suggests on THCB), and some decisions are operated by humans (ordering labs or MEDS or MEDS, or setting up people plans), and eventually some strategic decisions are made. In-depth research on large LLMs and advances in proxy AI have led many people, possibly including Satya Nadella, to suggest that Saas is dead. It’s not hard to imagine a new future where AI and agents run everything globally in the health system.
This leads to real problems for every participant in the healthcare ecosystem.
If you are buying an AI system, you don't know if the app or solution you are buying will be sucked by your own EHR or something that has been built inside your organization.
If you are selling an AI system, you don't know if your product is a feature of other people's AI, or if you are prompting your customers to want to develop skills rather than your tools. Worse, your prospects are barely punished, waiting to see if something better, cheaper will appear.
This is in a world where new, better LLM and other AI models are coming every few months.
I think the problem at the moment is that unless we have a clearer understanding of all these performances, there will be a lot of mistakes starting with no funding rounds anywhere, and the implementation of AI implementation has not achieved much. Reports like Bessmer's Sofia Guerra and Steve Kraus may help, providing 59 “work to do”. I'm just worried that no one will be too sure what the right tool for a job is.
Of course, I'm waiting for the robot overlord to tell me the correct answer.
Matthew Holt is a publisher of THCB